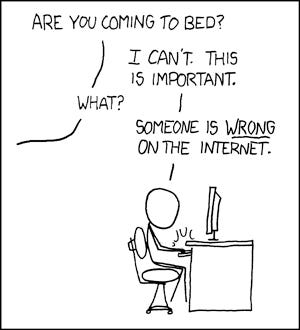
Data literacy training usually stops right where real life starts.1 Methods and case studies can be easily tidied up for the classroom, but that doesn’t always pay off in the muddy waters of real-world decision-making. What is usually missed is that data literacy is not just knowing what to do when analyzing data, it is also an intuition for knowing when something is wrong. Working with data is as much of an art as it is a science, and it requires nuance and skepticism to keep small missteps from becoming costly mistakes. From here, we’ll cover common pitfalls and warning signs to be aware of, as well as skills for investigating both your own data projects and those of others.
Cognitive biases are mental blind spots that arise when we trade accuracy for speed in our thinking. They are the natural result of our brains being wired to prefer patterns over noise - meaning everyone experiences them, and the best way to avoid them is to be aware of them. For example, confirmation bias is the tendency to look for information that supports our pre-existing opinions. This bias is in play most often when the data being analyzed is emotionally charged. This can occur when social norms, personal identity, or political risk are in play, where the stakes are high and maintaining objectivity is difficult. Another bias to consider is the availability heuristic, which is when events that are easier to recall - usually because they are surprising or extreme - are more likely to color our opinions, rather than those that are harder to recall. This is why anecdotal evidence often conflicts with data-driven decision-making. Quality data can correctly weight the frequency of ordinary versus extraordinary events, but our brains much prefer to amplify the extraordinary, resulting in a distorted perception of reality.
Similarly, our brains are prone to see cause-and-effect even when it may not exist. Real life is almost always too messy to precisely determine cause-and-effect relationships. For instance, higher education is closely connected to increased wealth, but the relationship is more complicated than it seems at first glance. Those who are highly educated are also more likely to have wealthy parents, meaning their personal wealth could be less about their education, and more about an inheritance or exposure to key financial principles at an early age. These events can also be reversed: those with greater financial stability are more likely to pursue higher education, since they have the resources to do so. Clear cause-and-effect relationships are not only rare, but also require the highest level of technical rigor to establish. This makes causation the most ambitious claim that can be made when working with data. The ideal strategy then, is to never assume causation in your own analysis, and to encourage others to be cautious in their interpretations as well.
The most obvious warning sign that something is wrong, is that the quality of your data isn’t what it should be. “Garbage in, garbage out” applies here. Is your dataset unusually small? You need a minimum of thirty data points to make any sort of statistical claim. Is there data that is obviously missing, whether that means some of your data points are missing a critical measurement, or that entire segments of the group you are analyzing are absent from the dataset? If you want your analysis to be as generalizable as possible, your data needs to be as complete as possible. For example, if your data was sourced from only one command, you cannot reliably apply your results to other commands as well.
Additionally, when the results from your analysis are unexpected, inconsistent, or too good to be true, it’s time to dig deeper. This doesn’t mean the outcomes can’t still be correct, but it does mean you have responsibility for understanding why they occurred. If your commander would question your results, you are better off questioning them yourself first. Lastly, complexity tends to appear more elegant than simplicity. The more expansive the dashboard, and the more obscure the mathematics, the more likely the analysis will catch our eye. However, if you find yourself thinking, 'it shouldn’t be this complicated', that's your sign to step back and assess whether the complexity is actually hiding a simpler, more effective approach to engaging with your data.
What guardrails can you put in place for your own work to avoid these pitfalls and warning signs? The first, and most important, is to begin your analysis with a hypothesis, and seek to disprove it rather than confirm it. This focus will help your skepticism stay fresh and will keep you wary of the cognitive biases we’ve discussed previously. Collaboration offers clarity as well. When your methods are transparent, your peers can sharpen and refine your processes and improve your awareness of your own blind spots. Here you have a choice: no analysis is perfect, so you can either (a) be transparent with your work throughout the process and learn about your mistakes within a trusted group of peers, or (b) keep your work close-hold to avoid criticism, and learn about your mistakes when presenting to your leadership or a broader audience. One of these is much more painful than the other.
When it comes to knowing if something is wrong with someone else’s work, we can apply the same processes from above. Is there transparency on the data quality and the methods used, or do these seem to be opaque no matter what questions are asked? If their work isn’t clear, the reason for that might be that they know it would fall apart with a more thorough review. Does the analysis come across as overconfident and over-extrapolated, with no room left for uncertainty? Someone appropriately confident in their work wouldn’t hesitate to discuss the uncertainty in their analysis and won't easily be persuaded to overstate the applications of their analysis, whereas someone less competent will try to skip to only the "good parts". Lastly, if it seems that they are applying data from your field of expertise incorrectly - such overlooking questions that only someone in your field might know to ask - then it is worth figuring out where the disconnect is. Again, this doesn’t mean that their conclusions are necessarily wrong, but it may mean that their efforts were not sufficiently informed by experts, leaving them unaware of specific issues that could affect the results.
As working with data requires as much art as it does science, honing your intuition is key to spotting mistakes that others may miss. This intuition is built through experience - ideally, that experience being someone else’s mistakes, and not your own. The availability heuristic is in your favor here, since stories are easier to recall than guidelines. Over time, as you gain experience with both successes and failures in working with data, your intuition will become a powerful tool for conveying nuance, calling out bluffs, and earning trust through accuracy.
If you’re a data nerd and somehow haven’t found XKCD yet, start with Thing Explainer and then check out his other books.